Our Research
This is a detailed description of our research interests. We begin with the basics and then cover more recent research interests.
The basics: Sensory coding by the vomeronasal system
To me (and probably to the rest of the lab as well), the most exciting questions about the brain concern its function as a computing device. A device that receives sensory information and produces a behavioral, physiological, or even mental output. Of course, numerous brain functions fall into this category. We study one of them: the processing of chemical information from other organisms, to guide behavioral or physiological outputs. For many animals, this is a very fundamental ability which is absolutely crucial for survival, as it guides responses to other organisms, whether conspecifics, potential predators, or prey.
The general problem we are getting it is described in a figure from this
hypothesis article.
An outline of our research. See
this for more details.
The idea is that organisms must derive information about other organisms to guide proper (or improper, we don't judge…) behavioral or physiological outputs. This requires that information about other organisms' traits (i.e. their species, sex, physiological state, individuality, and so on and on) will be reflected in the molecules that they emit. The brains' task then is to detect these chemical profiles, identify those that are informative for a specific aspect, and then use that information to act accordingly. In simple terms, much of our research involves recording neuronal activity (measuring stage "E" above) while presenting stimuli (collected from organisms as in "A") to animals and trying to figure out the complex processes that is represented by the single arrow in "D".
Vertebrates use more than a single system for chemosensation, and our focus is on the vomeronasal system (VNS), also known as the accessory olfactory system (AOS). See
this recent review for a description of the VNS. The VNS is present in most vertebrates and is prominent in rodents. One motivation for focusing on it is that we are quite confident about its role: to process cues from other organisms. This is not to be taken for granted as for many brain regions and systems there is no clear answer to the presumably simple question: what is their function?
Numerous studies (not ours) have shown that deficits in the VNS can lead to significant impairments associated with interactions with other organisms, whether conspecifics (from the same species) or heterospecifics (from other species). From a practical standpoint, there are advantages and disadvantages to studying this system. On the one hand, it is compact, both in size, and in the numbers of processing stages between sensory stimulus and hypothalamic neurons. The compactness facilitates a thorough analysis of the system, but there are also inherent challenges in studying it. One of these involves the system's physiology, and specifically its mode of sampling. Briefly, unlike the main olfactory system which continuously samples stimuli via breathing, VNs stimulus uptake is an active process that requires suction of solubilized stimuli into the vomeronasal organ in the nasal cavity. In some animals, such as horses (but not mice unfortunately), VNO stimulus uptake is externally visible.
We are not studying horses, but horses also have a VNS and when they activate it, it is visible. Photo credit to
Brandinian.
Our approach to induce stimulus update to the VNS involves electrical stimulation of the sympathetic nerve trunk. A few other labs in the world developed related preparations, each with their own pros and cons (In particular, see work from the Holy and Meeks labs). Our approach involves a delicate surgical procedure in which we implant a cuff electrode around the sympathetic nerve trunk, allowing us to repeatedly present stimuli to the VNS and measure responses to it. The preparation was first presented
here, and was described
here in more detail recently.
A schematic illustration of our preparation
Example of neuronal responses to social stimuli are shown below:
The image, taken from one of our recent
studies, shows responses from several AOB units, each of which is responsive to one of the two presented stimuli. Neurons shown on the left side seem selective to the stimulus donor's physiological state (in estrus, or not in estrus) whereas those on the right seem selective to an organism's strain (BC, for Balb/C or C57 for C57Black/6). One notable feature of AOB responses is their very long duration. We have recently
studied the temporal dynamics of AOB responses, and concluded that considering fine temporal dynamics does not significantly aid decoding of stimulus information. Regardless of temporal dynamics, note that for each trait (estrus, non-estrus, BalbC, or C57) we found at least one neuron that increases its firing rate. Note that each frame shows responses to one stimulus, urine, vaginal secretions (VS), and saliva. The figure highlights the fact that AOB neurons are indeed sensitive to the donors' identity and physiology, and that this information can be derived from multiple secretions.
Looking at these figures one might conclude that detecting relevant stimulus features is simple: you merely have to look at one of these neurons shown on the left to determine an animals' physiological state (estrus vs. non-estrus), or at one of the neurons on the right to detect its strain. But, this is a false conclusion. To illustrate, consider neuron 1 on the upper left side: this neuron responded to
a urine sample collected from estrus females and did not respond to another urine sample from non-estrus females. Yet, these are specific samples, from certain mice, at a certain age, at a certain reproductive status, a certain age, health status, and so on and on. Also, these are samples of one bodily secretion, urine. There is no basis to conclude that this neuron will respond selectively to any sample (urine, vaginal secretion, saliva, tear glands, etc…) from any estrus female, and not respond to any sample from a non-estrus female. In other words, based on this data, one should not confound selectivity to one feature in a stimulus set (and all sets are limited…) with general selectivity to the feature. In attempt to analyze this issue systematically we tested the ability of classifiers to extract behaviorally relevant information
from AOB neurons. Our conclusion is illustrated below (referring to the old tale of the blind men and the elephant):
We do not study elephants, but this image captures how information about other organisms is represented by groups of neurons (rather than single neurons). The image accompanied
this paper.
In words, we concluded that retrieving information about behaviorally relevant features requires pooling of information from multiple neurons. Why is this important? Because it is a departure from how we think of
pheromone based communication. Pheromones are often equated with social signals, but this is not justified. In fact, pheromones represent just one class of social signals, and are appropriate for eliciting stereotypic responses to stereotypic stimuli. This specifies not only the nature of the pheromone molecule, but also of the neuronal circuits required to detect and respond to it. For example, a pheromone is a suitable cue for eliciting an unconditional mating response between a male and a female, but not for conveying subtler information such as individuality, or specific physiological states.
These observations set the context for our research and analyses. And building upon this understanding of the system, we are interested in several other questions related to the function of the VNS.
Beyond the basics: Present interests and ongoing projects
Currently, we are interested in several questions related to VNS function. Taking a very crude approach, we can divide them to questions about plasticity vs. questions about basic functional aspects. However, the distinction is not clear cut, and the topics are interrelated.
Learning and plasticity in the VNS
Association of the VNS with innately meaningful cues might imply that experience and plasticity play only a minor role in its function. However, this is not the case. Responses to innately relevant stimuli could well depend on organisms' current state and previous experience. Furthermore, associative learning can endow a strong meaning to an initially neutral cue, resulting in a robust response to that cue. More generally, while all organisms engage in similar innate behaviors (eating, reproducing, competing for resources, evading danger…) the relevant stimuli that motivate or elicit such responses differ among species and among individuals within a species. In fact, the most fascinating aspect of innate behavior is perhaps the interplay between the common (almost everybody likes to eat) and the individual (different individuals enjoy different foods at different circumstances). Below is a description of some of the specific projects that we are currently pursuing under the general theme of plasticity.
Associative learning in an "innate" system
On the spectrum spanned by entirely innate vs completely learned, one extreme is a scenario in which a given stimulus elicits a hardwired rigid response. While no one has even claimed that the VNS is such a hardwired system, it was not known how easy it is to pair any pattern of activation with a learned, initially neutral response. In a recent project, we tested the ability of mice to learn to associate VNS activity with a learned
response. The major challenge was technical: activating the VNS without activation of the MOS. Activation by natural stimuli is not a good option since any chemical stimulus might affect sensory neurons in each of the two systems. Instead, we adopted an optogenetic approach in which we bypassed chemical stimulus and directly activated sensory neurons, or mitral cells of the VNS. Our conclusion was simple: it seems that the VNS can learn novel stimulus responses pairing as well as the main olfactory system.
The VNS can learn novel stimulus response pairings. Graphical abstract for our
paper.
Our study certainly does not rule out the option that some VNS mediated responses are hardwired, not does it tell us how much of VNS mediated behavior is actually learned. It does prove however that the system can learn, and thus that responses to cues with innate relevant can be modified. One of the caveats in our study is that stimulation patterns did not reflect natural stimuli or stimuli with some innate meaning. The more interesting question that we are setting to ask next is whether more natural response patterns can also be flexibly associated with novel responses and valence attributions.
State dependent plasticity
We all know that a given stimulus can elicit very different response depending on our current "state". For example, food may elicit varying responses depending on our state of hunger. Not only will we eat or not depending on our state, but even the valence we attribute to the smell or sight of food may be perceived as attractive or repulsive depending on our state. Likewise, sexual stimuli can elicit various responses depending on the state of "sexual satiety". Understanding the neuronal correlates and mechanisms of state dependent processing is a major endeavor in systems neuroscience. Naturally, we explore it in the context of the vomeronasal system, focusing on female subjects to ask how reproductive state modulates the representation of sexual cues. One project addresses the effect of pregnancy. This is particularly interesting in the context of the pregnancy block effect (Bruce Effect): When a pregnant female mouse is exposed to a strange male, there is a high chance that the pregnancy will be aborted. The really interesting question is why exposure to the father, the stud male, does not cause block. Together with our colleagues
Ian Davison in Boston University and
Steve Shea in Cold Spring Harbor Laboratories, we are collaborating to understand what changes in the mated female's brain to protect her from the stud male's odors.
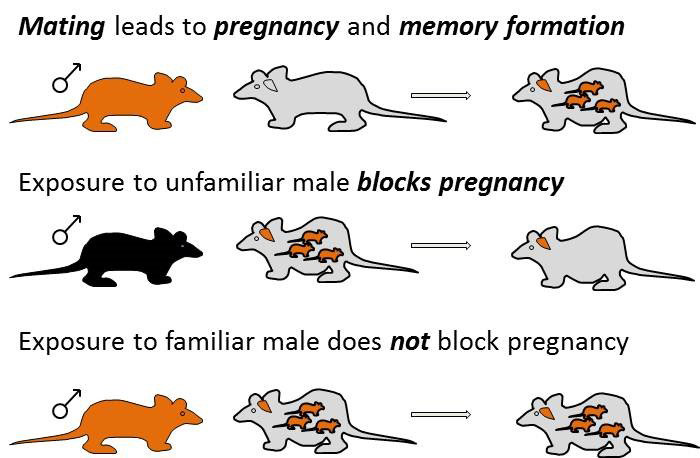
A schematic of the Bruce Effect
Another well-known example of state dependent processing in females undergoing estrus cycling is the modulation of sexual motivation during the course of the cycle. In this context, we study the effect of the different stages of the estrus cycle on responses to sensory cues.
A schematic of state dependent sexual responses in female mice
A general feature that is beginning to emerge from these studies is that stimulus representations at the level of the AOB are relatively robust to such state dependent processing. This leads us to suspect that more dramatic effects might be found downstream to the AOB, for example in the Amygdala. This is consistent with
work performed with Joe Bergan at the lab of Catherine Dulac.
Computational aspects of VNS function
We would ultimately like to understand how to read information about the stimulus from neuronal activity. As described above (see the elephant cartoon), information is distributed over a population of AOB neurons. One central question is what do AOB neurons represent. In other words, which stimulus features is their activity informative about. A different, but related class of questions concerns the temporal aspect – namely, how is stimulus information represented in time.
Tuning profiles of AOB neurons
There is something weird about the way the vomeronasal system is wired. Wouldn't you expect a clear organization in a system that is supposed to detect well-defined cues? Looking at the AOB gives the impression of a mess: just look at this image of the olfactory bulb by Ramon Y Cajal:
Ramon y Cajal, S. (1901). Textura del lóbulo olfativo accesorio. Trab. Lab. Invest. Biol. 1, 141–149.
The orange boundary encloses cell bodies of mitral tufted cells of the main olfactory bulb. The red boundary shows cell bodies of mitral tufted cells of the accessory olfactory bulb. While both neuron classes are the output neurons, there are clear differences. Main olfactory bulb mitral cells are larger, more uniform in their position, and perhaps most importantly, they send dendrites to sample information from a single glomerulus. In contrast, accessory olfactory bulb mitral tufted cells send their dendrites to multiple glomeruli. Closer inspection shows that individual neurons can sample inputs from multiple glomeruli (between 1 and 10). This implies that whereas MOB mitral cells roughly inherit the tuning properties of single glomeruli (the glomeruli they sample input from), receptive fields of AOB mitral tufted cells may be considerably more complex. It is very hard to deduce receptive properties from connectivity patterns, but the images shown in the figure above, and others like it, suggest that AOB mitral tufted cells may have complex receptive profiles. But what are these response profiles? One possibility is that AOB neurons sample specific combinations of molecules that when present together unequivocally report some feature. Another is that AOB neurons sample glomeruli entirely randomly. While some evidence (from
Shlomo Wagner's work in the Dulac lab) suggests that sampling is not entirely random, the overall picture is not clear. Ideally, one could record from individual neurons while presenting numerous combinations of molecules and measuring the neuronal responses. If for each AOB mitral tufted cell, we knew how it responded to any combination of molecules, and what each stimulus combination implies about the organism that emitted it, then we would have a very good understanding of the information that the AOB sends to its downstream targets. But this is not feasible… and thus we have to make do with simpler, and more indirect ways to learn about the receptive fields of these neurons. Given our current experimental abilities, the most productive approach is to carefully select stimulus sets and then to examine which stimulus features are best represented by neuronal activity. For example, using a data set that contains stimuli from two strains of male and female mice, and predator stimuli, we can ask whether there is an overabundance of neurons representing features. The example below shows a response matrix of 263 AOB neurons to these five different stimuli. For visualization purposes, responses were normalized and non-significant responses have been set to 0. Although this is a small stimulus set, it allows definition of various categories. For example, we can define a female specific neuron as a neuron whose responses to the two female stimuli are larger than all other stimuli. We can then ask if these neurons are more frequent than expected by chance. On the right panel, we see the expected distribution of the number of neurons in each of these categories (in blue), and the actual number of neurons observed in the data, in red. For three of the categories in this dataset, there is a clear overabundance of category specific neurons, these are "female specific" neurons, "male specific" neurons, and "mouse specific" neurons. Note that there is no overrepresentation of "strain specific" neurons. The quotation marks are important: they indicate that we do not really believe these are universal female neurons, but rather that in this specific dataset, they can be classified as such. The reasons we do not think these are universal male or female specific neurons is that when we examine larger stimulus sets, many of these categories break down.
Response matrix of ~250 neurons to 7 different stimuli (left) and representation of categories (right). Blue curves show the expected (bootstrapped distributions) while the red line shows the actual number of neurons conforming to each category.
Regardless of whether these are male or female specific neurons, the frequency of each of these types of responses types can tell us something about how AOB neurons represent stimulus information. This is especially true when we these responses are evaluated in conjunction with information about the chemical constituents of the stimuli, and responses observed at the level of VSNs. This is an effort that we are leading with our collaborators in the groups of Prof. Marc
Spehr and Pavel
Stopka. In addition, combining this approach with our interest in plasticity as described above, we can ask whether the representation of such categories changes as a function of experience.
Tuning profiles of AOB neurons
Also related to coding, but addressing a different question concerns the temporal features of the responses. More specifically, what role does the temporal domain play in stimulus coding? One line of research concerns response timing relative to stimulus onset. Another involves more global rhythmic patterns.
Temporal aspects of VNS activity
Temporal coding (which can mean different things to different people), is a popular theme in neuroscience. A distinction is made between temporal and rate coding, and while almost everyone agrees that this is not a clear dichotomy, it is valid to ask about the relevant time scales that convey information about the stimulus. In the main olfactory system, there is a natural temporal metric: breathing. The ongoing process of breathing couples stimulus delivery to inhalation and one may ask if the timing of neuronal activity with respect to this cycle is informative. Studies using various approaches from several labs indicate that the answer is positive. That is, consideration of neuronal activity with respect to the breathing cycle provides more information than is available by simply counting all spikes during an entire breathing cycle. In the VNS, there is no obvious ongoing cycle, but this does not preclude the relevance of temporal coding. If one considers the vomeronasal analogue of the sniff to be stimulus uptake, then we can ask whether neuronal response dynamics relative to the initiation of uptake is informative.
To address this question, we have
recently examined whether consideration of "fine" temporal dynamics provides more information that does consideration of the number of spikes generated within a broad window (e.g. firing rate over a long time window). In the figure below, we show the success of various temporal scenarios (which differ in the duration and the number of temporal windows) in decoding stimulus information. Each of the decoding schemes has its advantage and its costs. Perhaps the simplest scheme is to count the spikes since stimulus uptake. Using this approach (cumulative single window, green line in panel B), for this stimulus set, stimulus identity can be detected in ~70% of the cases after about 5 seconds. To achieve the highest performance (~83%) using this scheme, it is required to sample for about 20 seconds. Slightly better performance can be obtained by considering a smaller window that begins after stimulus onset (red lines in panel C).
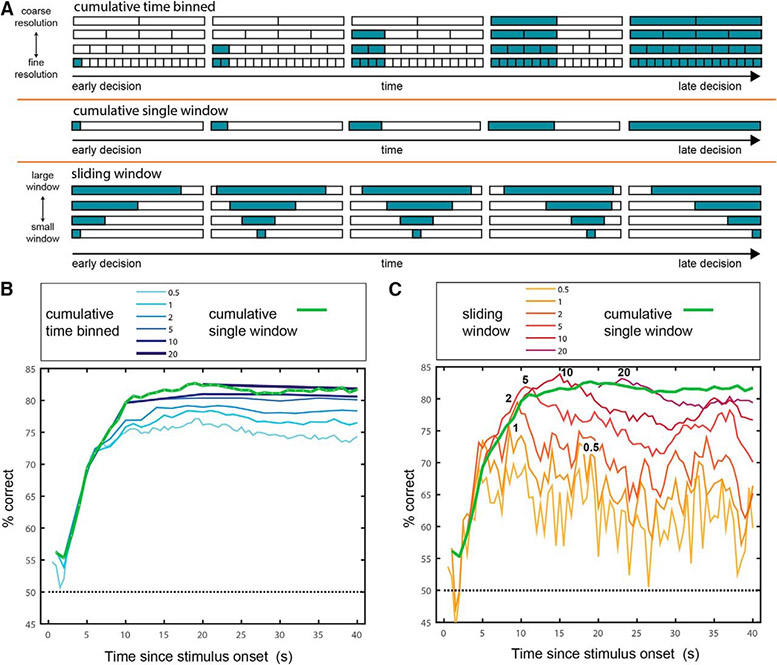
All the temporal windows above require knowledge of the timing of stimulus onset. While it is very feasible that this information is available to neuronal decoders of AOB activity, due to the nature of VNO stimulus uptake, sampling is very variable temporally. We thus asked if AOB responses can be decoded without knowing the time of stimulus onset. We found that this scheme, which we called time invariant decoding, can provide above-chance performance, for windows as small as 0.5 s. We also found that multiple neurons are required for this decoding schemes. In other words: multiple neurons are required not only to overcome variability in the properties of the stimulus, but also in the temporal domain of the responses (Panel A below). Panel B below illustrates the tradeoff between integration window duration, number of neurons in the population, and the decoding performance. Expectedly, longer neurons and longer integration windows result in better decoding.
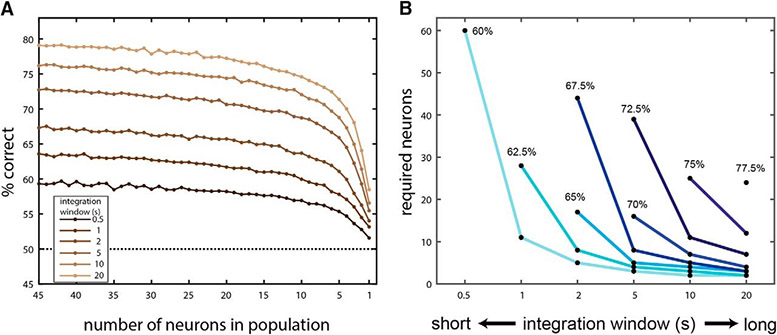
Putting the details aside, these analyses suggest that fine temporal dynamics is not a key feature of AOB activity. This makes sense to us, because VNS stimulus uptake is inherently a slow process. Why is uptake slow? Ultimately, this involves the dynamics of the stimuli, which do not change rapidly. Unlike say the pressure waves that fluctuate at frequencies of ~10K Hz to create sound, the composition of molecules in urine or other bodily secretions does not change rapidly and there is no benefit in very rapid sampling (which in this case is also constrained by the nature of the stimulus). If stimuli change slowly, then slow firing rates suffice to convey the information (fast rates are required for transmission of quickly changing stimuli), and if slow firing rates can convey the relevant information, there is no benefit to employ costlier firing rates. Indeed, AOB neurons do not exhibit very high firing rates, and given the nature of the stimulus and its uptake, this probably does not impose a bottleneck in the transmission of chemosensory information to deeper brain regions.
Oscillations in the OB
As mentioned above, our analyses of VNS temporal dynamics were inspired by studies on the main olfactory system. A related set of studies in the main olfactory system examines the roles of oscillations, and particularly, population oscillations as expressed by the local field potential (LFP) signal. There are various patterns of main olfactory system LFP oscillations, each likely involving a distinct set (or sets) of neurons. This implies that the different patterns vary in their waveform characteristics, spatial loci, and their correlation with particular functional aspects. While it is still not clear what function, (if any…), these oscillations serve, they can probably tell us something about the processes that underlie chemosensory information processing. Several studies looked at LFP oscillations in the AOB, but none were clearly related to stimulus delivery. Recently, we have found such oscillations in our own recordings in anesthetized mice, and these are very similar to oscillations described by Lanuza and colleagues
in vivo. Currently, we are working to better characterize these oscillations and their relationship to stimulus delivery. In parallel, with our colleagues from the Spehr group, we are trying to understand the mechanisms underlying these oscillations.
Stimulus-induced LFP oscillations in the AOB. (A) Simultaneous LFP recordings from 32 sites (~20 s; 1–300 Hz; 50 Hz 'hum' removed). Plot reflects the spatial organization of recording sites (bottom sites deeper, top sites more superficial). The array's center is located within the AOB mitral cell layer. The black vertical 'bar' in each panel is an artefact from sympathetic nerve stimulation. Note the change in LFP power in those channels located in the AOB. While only one example is shown, oscillatory patterns are repetitive within and across recordings. Instantiations differ in temporal details, but general features are consistent. (B) Detailed view of one channel (gray) on expanded temporal coordinates. Note the slower initial modulation, followed by faster oscillations. (C) Spectral analysis of the signal in (B) (1 s Hamming window, 0.8 overlap). Note apparently discrete frequency jumps, beginning at ~5 Hz and ending at ~15 Hz. |